Promising potential for comprehensive health monitoring
Human Activity Recognition (HAR) enables the continuous monitoring of static as well as dynamic behaviors in different environmental conditions. In combination with the continuous measurement of vital signs, there is great potential for the healthcare sector, e.g. for the early detection of diseases and the precise monitoring of their progression. Lukas Boborzi, PhD Student at the Ludwig-Maximilians University of Munich, together with the German Center for Vertigo and Balance Disorders, conducted a study that explored an approach by utilizing the in-ear vital signs sensor c-med° alpha for Human Activity Recognition.
Human Activity Recognition (HAR) in healthcare
HAR is now widely applied in different fields such as industries, sports, and healthcare. Especially in the healthcare sector HAR monitoring presents a chance to gather ecologically valid information about a patient’s condition in everyday environments and allows for the detection of rare and fluctuating events that are often missed in short-term clinical assessments. Thus, this technology has a high potential for disease detection in preclinical stages and monitoring of disease progression. It also allows for evaluation of intervention effects and fall detection.
In-ear vital signs monitor combined with HAR: Potential benefits
The ear, in contrast to the lower extremities or the trunk – both locations often used in other approaches – apparently has several unique advantages for connecting an HAR device. For one the head remains exceptionally stable during various movements. This provides a reliable locus for low-noise identification and differentiation of different bodily activities.
Secondly, beyond motion, the ear is an ideal location for assessing a person’s physical and health status, as optical in-ear sensors can reliably capture vital signs such as heart rate, body temperature, and oxygen saturation in the ear canal. Therefore, the ear stands out as a promising candidate site for wearing a single integrative sensing device, facilitating comprehensive continuous monitoring of a person’s activity and health status in everyday life. Combining vital signs monitoring and HAR allows for the observation of vital sign changes and fluctuation in the context of behavior. This provides an opportunity to correlate vital signs with activity and establish individual baselines to identify abnormalities. It also allows to detect behavioral patterns and habits impacting health.
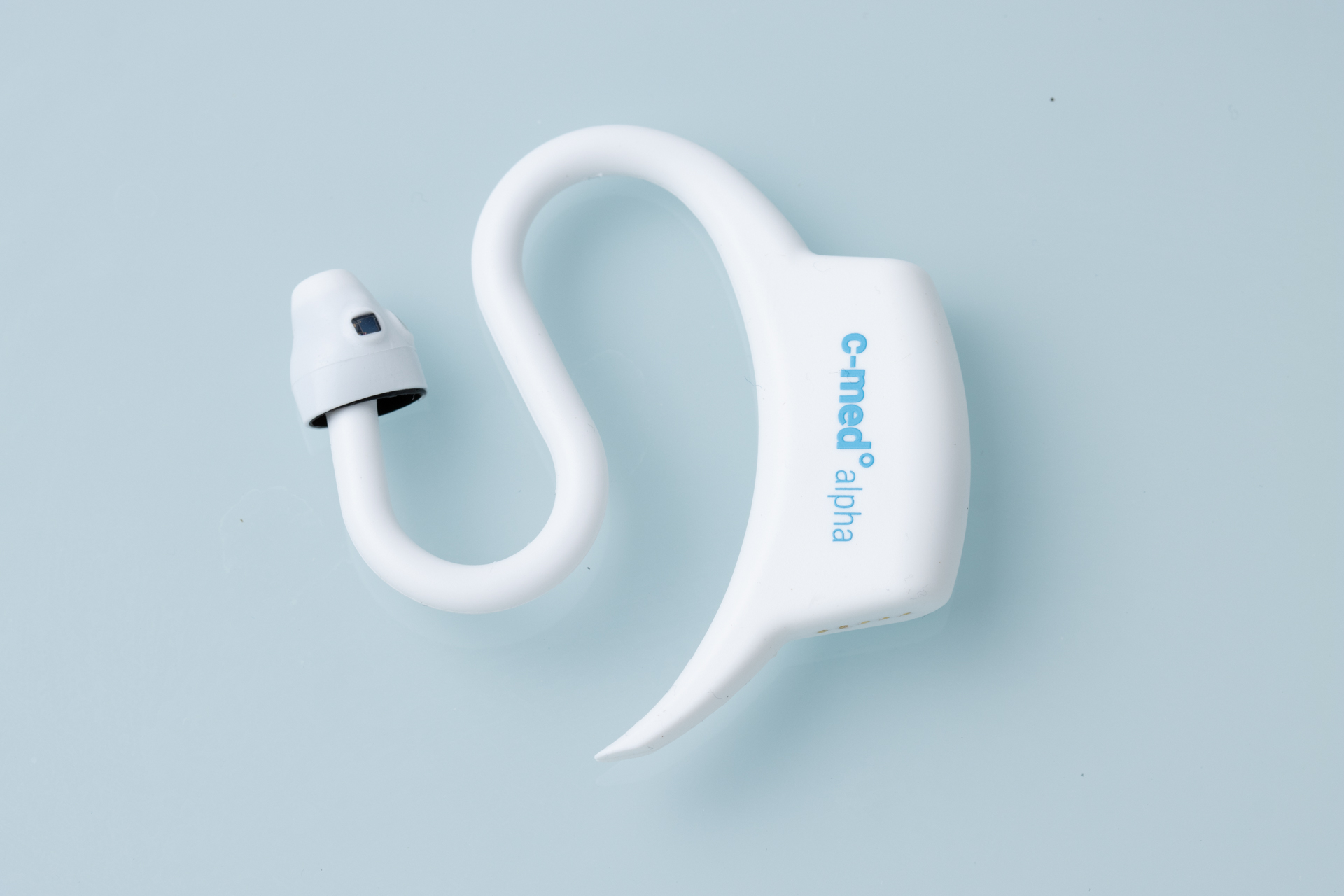
In-ear sensor c-med° alpha, a class IIa medical measuring device that generates continuous data streams of three important vital signs: Core body temperature, heart rate, and blood oxygen saturation (SpO2).
Study setup: Participants and methods
The objective of this study was to investigate the potential of the c-med° alpha to classify various human activities. In fifty healthy individuals between 20 and 59 years1 daily activities such as lying, sitting, standing, walking, running, and stair walking were recorded and labeled in a free-living environment while wearing the c-med° alpha.
To classify these activities, they were manually labeled and subsequently machine learning algorithms were employed, adopting a two-fold strategy: shallow machine learning models utilizing interpretable features or parametrizations of movement (e.g., movement amplitude and variance) and state-of-the-art deep learning algorithms specifically designed for HAR.
Results: Activity clustering and classification
For shallow learning, the acquired three-dimensional motion time series from the sensor (accx, accy, accz) were segmented by using the sliding window technique employing various sizes of non-overlapping windows (i.e.: 0.5, 1, 2 s) to determine an optimal configuration. After segmentation, a set of time-domain and frequency-domain features established for time series analysis of physiological signals were computed per segment. In addition, the Pearson and Kendall correlation coefficients were computed for every combination between motion axes resulting in a total number of 64 features. Using these features in a 2D-UMAP plot resulted in well discernable clusters for most activities (see Figure 1).
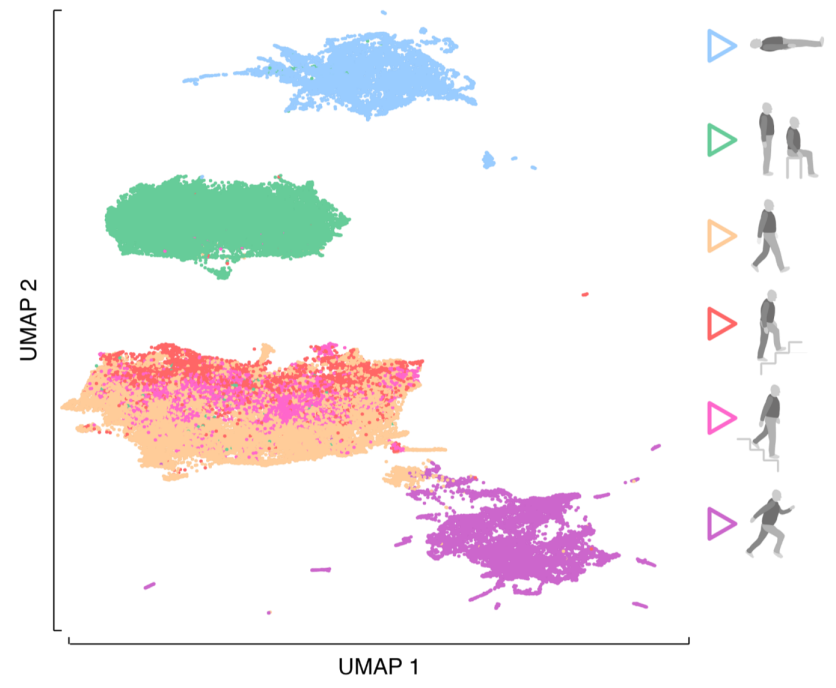
Figure 1: Natural clustering of different activities using 64 statistical features.
For pre-evaluation, a set of eight standard machine learning models were trained. Shallow learning models yielded at best an average classification accuracy of 95%. It was possible to almost perfectly identify and differentiate between the activities lying, sitting/standing, and running but there were still some difficulties in distinguishing between the activities walking, ascending and descending stairs.
Two deep learning models specifically designed for the task of HAR on wearable motion signals were considered. The best performing model was the DeepConvLSTM architecture. It combines a convolutional neural network with long-short-term memory recurrent network (LSTM). The best performing deep learning model was DeepConvLSTM with a 2s window size. This classifier yielded a very high performance and was able to identify activities with an average accuracy of 98% (see Figure 2).
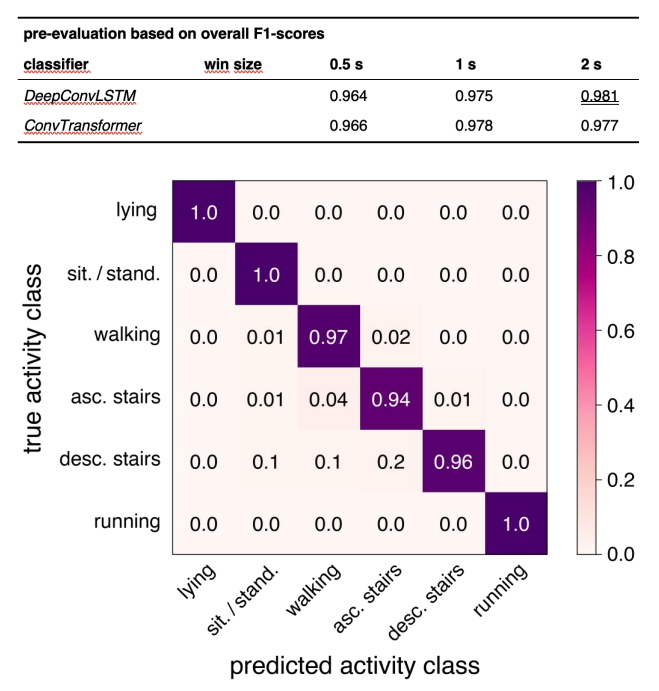
Figure 2: Confusion Matrix of the best performing deep-learning model (DeepConvLSTM, 2s win).
Summary: Practical use of vital signs monitoring combined with HAR and future developments
This study showed that when deep-learning algorithms are applied and possibly integrated in future software iterations the cosinuss° c-med° alpha with its build-in vital sign sensors is a good option for human activity recognition and long-term patient monitoring. An example of how this could look in the future is shown in figure 3.
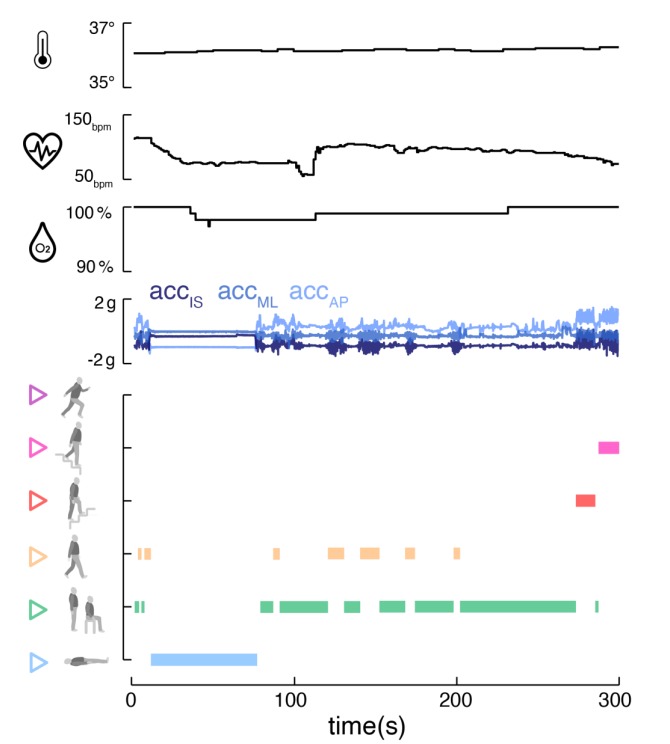
Figure 3: Example of combined HAR and vital sign monitoring during different activities.
As mentioned at the beginning, HAR technology has significant potential in clinical and care settings. It offers the opportunity to study the impact of physical activity on vital signs and provides a better understanding of daily activity patterns that influence health status. In addition, the integration of HAR with vital signs measured in the ear promises to shed light on the reciprocal relationships between diseases and vital signs and activity levels.
Future investigations resulting from this study could delve deeper into gait analysis. This expanded focus could facilitate various clinical applications, such as early diagnosis of certain conditions (even before the onset of visible symptoms), tracking disease progression, monitoring interventions using digital biomarkers, and risk stratification (especially for fall risk assessment). Such developments pave the way for valuable healthcare interventions in the future.
Link to the publication
Boborzi, L.; Decker, J.; Rezaei, R.; Schniepp, R.; Wuehr, M. Human Activity Recognition in a Free-Living Environment Using an Ear-Worn Motion Sensor. Sensors 2024, 24, 2665. https://doi.org/10.3390/s24092665